Machine Translation: Poisonous & Evil Rubbish?
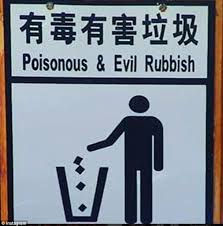
Timothy Hunt said that computers will never replace translators, but translators who use computers will replace translators who do not[1]. This seems to be the widespread perception in the translation industry, especially considering that, according to estimations, the use of translation technology has made it possible for one translator to carry out the work of six[2]. In fact, the general consensus both among trainers and industry stakeholders is that in order to succeed in this field and translators are actually required to master technology.
We can't deny that the development of CAT tools has revolutionised our sector and, personally, I do not know of any translators who live as hermits, with no WI-FI connection or access to CAT tools, translating exclusively from paper-based dictionaries and glossaries. We might complain about aspects of technology and the way it is affecting our profession in terms of rates and demands from our clients (for instance, in the case of MT post-editing, fuzzy matches etc), but we cannot put technology on trial and start a witch-hunt. Technology helps us: spell-checkers are technology, TMs are technology, Google and online dictionaries are technology. I am fairly positive that no translator is advocating getting rid of these tools and types of technology! Things get trickier, though, if we talk about MT.
MT continues to be a controversial topic in the industry, but it is undeniable that it has brought along a paradigm shift. There have been several studies focusing on the uptake of MT but results are not clear-cut. Often, what studies reveal about MT seems to be in conflict with what translators say about it on Facebook forums, blogs, or in real life for that matter. Some studies even claim that translators are wary of using MT for a series of reasons, and that they have a negative bias against it, and are not always accurate or impartial when they criticise MT. In contrast, a lot of academic studies focusing on MT evaluation seem to focus mainly on the silver lining of this technology.
Nonetheless, even the European Master's in Translation (EMT) expert group, a group set up by the Directorate General for Translation of the European Commission (DGT) with the goal of improving the quality of translator training by implementing a European reference framework for Master’s programmes in translation in the EU, stresses the importance of technology, post-editing, and being familiar with MT, so we cannot turn a blind eye and hope MT will simply disappear off the face of the Earth.
So far, Statistical Machine Translation (SMT), in which translation knowledge is built from corpora of naturally occurring language, has dominated the market. However, a new paradigm is currently at its initial deployment stages: Neural Machine Translation (NMT). According to some studies, it seems to be performing better than SMT, producing a language that is more fluent and more grammatical, which would ease the post-editing efforts.
Just last year, Google announced the launch of its new AI system (Google Brain), based on deep neural networks, which would ultimately replace Google Translate as we know it. This system appears to have shown "overnight improvements roughly equivalent to the total gains the old one had accrued over its entire lifetime"[3], and it has sparked enthusiasm both among industry stakeholders and developers. DeepL, another NMT system, developed initially from Linguee, seems to provide promising results as well. In fact, it appears to be more nuanced than Google Translate and produces fewer errors of tense, and has a better understanding of idioms, making the translation more readable and smooth[4] (this, of course, according to either its developers or academics who have tested and studied these NMT systems).
However, fear not: NMT will not be replacing flesh and bone translators anytime soon! Some studies highlight that NMT does not perform well in the case of longer sentences, with more than 60 words. It also seems to skip some of the source content, which is not translated in the output. This is, obviously, a serious issue that cannot be overlooked.
According to Ketzan[5] "Technology may have put us on the moon, but machine translation has the potential to take us farther, across the gulf of comprehension that lies between people from different places". That may be the case, however, we cannot expect MT to match or surpass human translators, since they would be required to have real-world knowledge to do so. Many MT developers and industry stakeholders (aka not translators) claim that MT is revolutionary. Regardless of what our stance is on this issue, we, as translators, cannot be reactionary. Even if at a certain point in the future MT will become so advanced that it will be able to produce smooth, natural-sounding texts in technical, repetitive fields, it will never replace human translators in sectors that require creativity, in the localization industry, in literature or tourism, and so on.
The "One Hundred Year Study of Artificial Intelligence"[6] concluded in their 2016 that the rise of AI will have a similar effect on cognitive jobs as the automation revolution had on the manufacturing sector. Therefore, in the medium to long term, the need for retraining and adapting (creating and offering new services and solutions, using technologies to our advantage to enhance productivity) will be imperative in order to mitigate the effects of AI on the job market. If we are proactive instead of reactive, we will never be replaced.
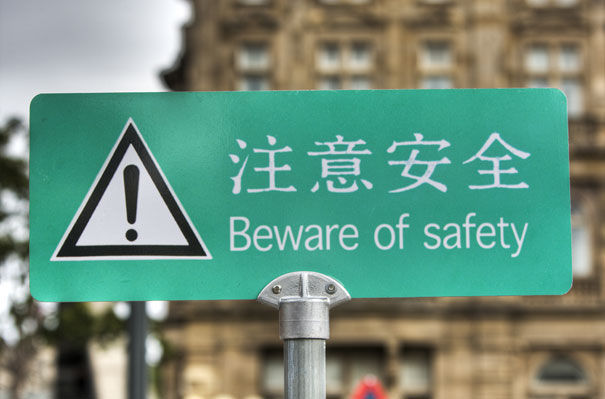
Further Reading:
1. Sofer, M. (1999). The Translator's Handbook. Rockville: Schreiber Publishing.
2. Sin-Wai, C. (ed.) (2015). The Routledge Encyclopedia of Translation Technology. Oxon: Routledge
3. Lewis-Kraus, G. (2016). The great A.I. awakening. The New York Times. [online]. Available from: https://www.nytimes.com/2016/12/14/magazine/the-great-ai-awakening.html?mcubz=0
4. Coldewey, D., and Lardinois, F. (2017). DeepL schools other online translators with clever machine learning. Tech Crunch. [online]. Available from: https://techcrunch.com/2017/08/29/deepl-schools-other-online-translators-with-clever-machine-learning/
5. Ketzan, E. (2007). Rewriting Babel: Copyright and the Future of Machine Translation Online. Tulane Journal of Technology and Intellectual Property 9, pp. 205-234.
6. Stone, P., Brooks, R., Brynjolfsson, E., Calo, R., Etzioni, O., Hager, G., Hirschberg, J., Kalyanakrishnan, S., Kamar, E., Kraus, S., Leyton-Brown, K., Parkes, D., Press, W., Saxenian, A., Shah, J, Tambe, M. and Teller, A. (2016). Artificial Intelligence and Life in 2030. One Hundred Year Study on Artificial Intelligence: Report of the 2015-2016 Study Panel. Stanford: Stanford University. [online]. Available from: http://ai100.stanford.edu/2016-report